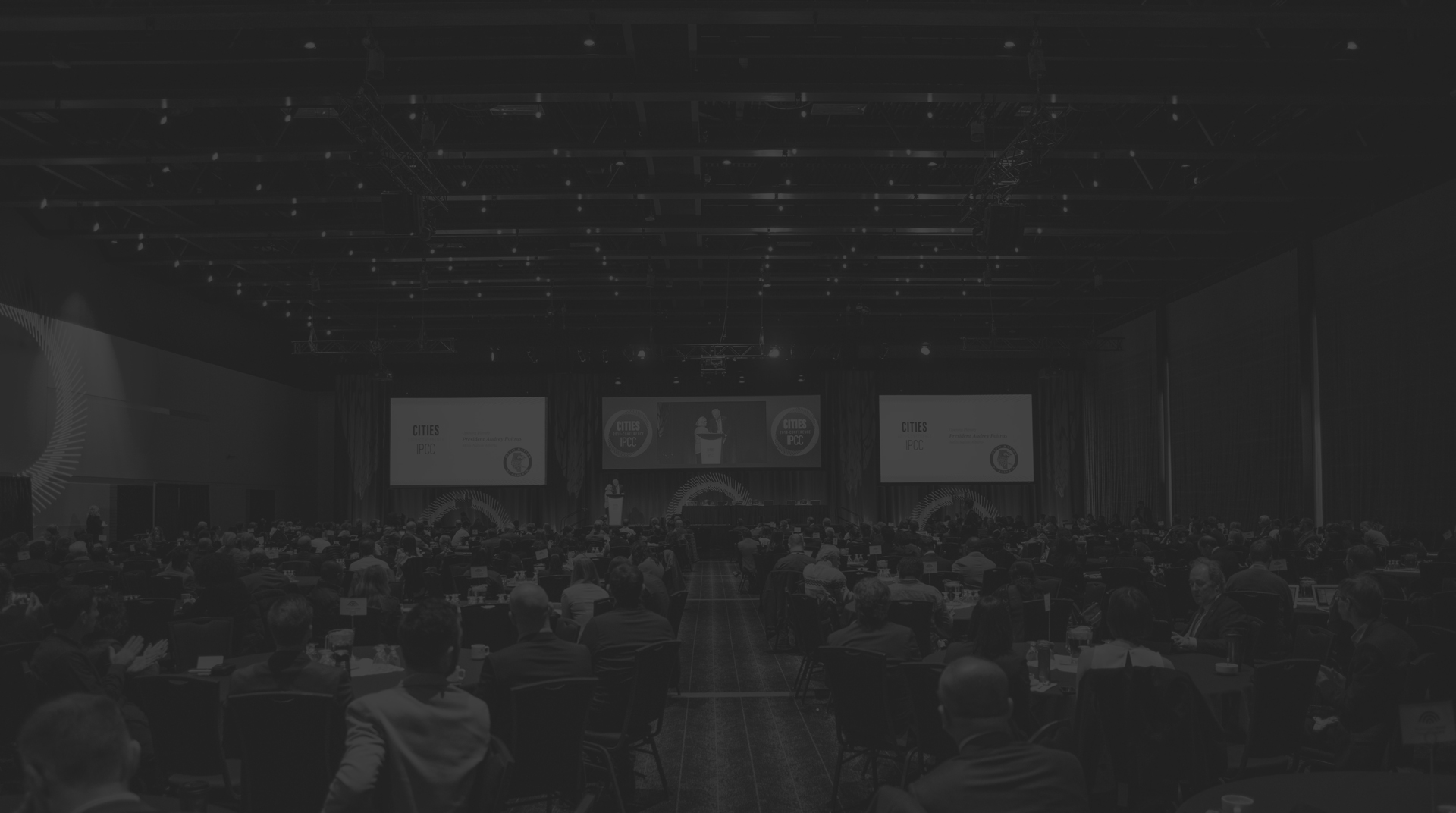
Event on: November | 11-12 | 2019
International Conference on
Big Data Analytics and Data Science
Theme : Building the Data-Driven Future of Big Data

Las Vegas, USA
Automated thinking is the data performed by machines or software demonstrated by machines, in contrast to the natural intelligence displayed by humans and other animals. AI examination is amazingly particular and focus, and is essentially isolated into subfields that a great part of the time hatred to chat with each other. It solidifies Artificial Creative Ability, Artificial Neural structures, Adaptive Systems, Cybernetics, Ontologies and Knowledge sharing.
Big data analytics probe and analyse huge amounts of data to i.e., big data - to uncover hidden patterns, unknown co-relations, market trends, customer preferences and other useful information that can help organizations make more-informed business decisions. Operate and carry by specialized analytics systems and software, big data analytics can lay the way to various business benefits, including new revenue opportunities, more effective marketing, improved operational efficiency, competitive advantages and better customer service.
With advances in technologies, nurse scientists are increasingly generating and using large and complex datasets, sometimes called “Big Data,” to promote and improve Health Conditions. New strategies for collecting and detailed examination large datasets will allow us to better understand the biological, genetic, and behavioural underpinnings of health, and to improve the way we prevent and manage illness.
Big Data is the name given to huge amounts of data. As the data comes in from a variety of sources, it could be too diverse and too massive for conventional technologies to handle. This makes it very important to have the skills and infrastructure to handle it intelligently. There are many of the big data solutions that are particularly popular right now fit for the use
Big data has increased the demand of information management so much that most of the world’s big software companies are investing in software firms specializing in data management and analytics. According to one rough calculation, one-third of the globally stored information is in the form of alphanumeric text and still image data, which is the format most useful for most big data applications. Since most of the data is directly generated in digital format, we have the opportunity and the challenge both to influence the creation to facilitate later linkage and to automatically link previously created data. There are different phases in the Big Data analysis process and some common challenges that underlie many, and sometimes all, of these phases.
Cloud computing is the delivery of computing services—servers, storage, databases, networking, software, analytics, and more—over the Internet (“the cloud”). Cloud computing relies on sharing of resources to achieve coordination and economies of scale, similar to a public utility. Companies offering these computing services are called cloud providers and typically charge for cloud computing services based on usage.
The uncertainty of a calculation indicates the aggregate time required by the system to rush to finish. The many-sided quality of calculations is most generally communicated using the enormous O documentation. Many-sided quality is most usually assessed by tallying the number of basic capacities performed by the calculation. What's more, since the calculation's execution may change with various sorts of info information, subsequently for a calculation we normally use the most pessimistic scenario multifaceted nature of a calculation since that is the extended time taken for any information size.
The data architect and data engineer work in tandem – conceptualizing, visualizing, and then building an Enterprise Data Management Framework. The data architect visualizes the complete framework and creates the blueprint, which the data engineer uses to build the “digital framework.”
The data engineering role has recently evolved from the traditional software-engineering field. Recent Enterprise Data Management experiments have proven beyond doubt that these data-focused software engineers are needed to work along with the data architects to build a strong Data Architecture. Between 2013 and 2015, the growth of data engineers was around 122 percent in response to a massive data industry need.
Data mining is the process of discovering patterns to extract information with an intelligent method from a data set and transform the information into a comprehensible structure for further use. Data mining is the detailed examination step of the "knowledge discovery in databases" process. These applications relate Data mining structures in genuine cash related business territory examination, Application of data mining in positioning, Data mining and Web Application, Engineering data mining, Data Mining in security, Social Data Mining, Neural Networks and Data Mining, Medical Data Mining, Data Mining in Healthcare.
Both data science and machine learning are rooted in data science and generally fall under that category. They often intersect or are confused with each other, but there are a few key contrasts between the two. The major difference between machine learning and data mining is how they are used and applied in our everyday lives. Data mining can be used for a variety of purposes, including financial research, Investing, sales trends and marketing. Machine learning visible form of the principles of data mining, but can also make automatic correlations and learn from them to apply to new algorithms.
Information representation is seen by numerous orders as a present likeness visual correspondence. It is not held by any one field, yet rather discovers translation crosswise over numerous. It covers the arrangement and investigation of the visual representation of information, indicating "data that has been dreamy in some schematic structure, including attributes or variables for the units of data".
In computing, a Data Warehouse (DW or DWH), also known as an Enterprise Data Warehouse (EDW), is a system used for reporting and data analysis and is considered a central component of business intelligence. Data Warehouse or Enterprise Data Warehouse is central repositories of integrated data from one or more disparate sources.
With pervasive sensors continuously collecting and storing massive amounts of information, there is no doubt this is an era of data deluge. Learning from these large volumes of data is expected to bring significant science and engineering advances along with improvements in quality of life. However, with such a big blessing come big challenges. Running analytics on voluminous data sets by central processors and storage units seems infeasible, and with the advent of streaming data sources, learning must often be performed in real time, typically without a chance to revisit past entries. “Workhorse” signal processing (SP) and statistical learning tools have to be re-examined in today’s high-dimensional data regimes.
Natural Language Processing :
Natural language processing (NLP) is a subfield of computer science, information engineering, and artificial intelligence concerned with the interactions between computers and human (natural) languages, in particular how to program computers to process and analyze large amounts of natural language data.
IoT and edge computing applications :
The internet of things, or IoT, is the network of physical devices interrelated computing devices, mechanical and digital machines, objects, animals or people that are provided with unique identifiers(UIDs) and the ability to connect, collect and exchange data or transfer data over a network without requiring human-to-human or human-to-computer interaction.
Predictive analytics encompasses a variety of statistical techniques from data mining, predictive modelling, and machine learning, that analyze current and historical facts to make predictions about future or otherwise unknown events.In business, predictive models exploit patterns found in historical and transactional data to identify risks and opportunities. Models capture relationships among many factors to allow assessment of risk or potential associated with a particular set of conditions, guiding decision-making for candidate transactions. The defining functional effect of these technical approaches is that predictive analytics provides a predictive score (probability) for each individual (customer, employee, healthcare patient, product SKU, vehicle, component, machine, or other organizational unit) in order to determine, inform, or influence organizational processes that pertain across large numbers of individuals, such as in marketing, credit risk assessment, fraud detection, manufacturing, healthcare, and government operations including law enforcement.
Data-driven Analytics and Business Management:
In business, you constantly have to make decisions — from how much raw material to order to how to optimize retail traffic for changing weather. In days gone by, you might have consulted the person who had been around the longest for their best guess; for a more scientific approach, you might have also looked at sales records. Today, companies are finding that the best answers to these questions come from another source entirely: large amounts of data and computer-driven analysis that you rigorously leverage to make predictions. This is called data-driven decision making (DDDM).